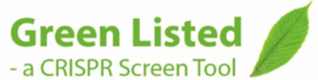
This post was contributed by guest blogger Fredrik Wermeling, leader of a research group at the Centrum for Molecular Medicine (CMM), Department of Medicine, Solna, Karolinska Institutet, in Sweden.
It can be very time consuming to design 5 guide RNAs (gRNAs) targeting each of the 1000 genes you’d like to investigate in your next CRISPR screen. Luckily, the Green Listed software can help you do just this, probably in less than a minute (1).
Green Listed is a new software tool used to design gRNAs for custom CRISPR screens targeting a (long or short) list of genes provided by the user. This approach is useful for several applications as will be discussed below. The software could also be used as a simple tool to rapidly find one or a few gRNAs suggested to be effective by world class CRISPR labs.
Check Out This Video to See How Green Listed Works at a Glance!
CRISPR knockout screens: Full genome vs. custom screens
Full genome CRISPR knockout screens have been used in several settings, and are fantastic tools for unbiased discovery. With these screens, the investigator generates a cell population where all genes are knocked out at the population level, but each individual cell only has a maximum of one gene knocked out. In this genetically heterogeneous population, cells behaving differently (e.g. different growth properties, migration abilities, or other phenotypes that can be efficiently measured at the single cell level), are separated by, for example, cell sorting. The separated cells are subsequently sequenced by next generation sequencing to identify which gRNAs are differentially expressed in the separate groups and, as a consequence, which genes are good candidates for being involved in the studied phenotype.
For robustness of a screen, each gene is not targeted by only one gRNA, instead many different gRNAs are used for each gene. At the moment, 13 full genome SpCas9 knockout libraries designed by different academic labs, targeting mouse, human, Drosophila melanogaster, and T. gondii are available through www.addgene.org/crispr/libraries/. Additional libraries are also available from commercial vendors. These libraries usually contain 4-12 gRNAs/gene, which results in libraries containing up to around 200,000 different gRNAs. It has been suggested that researchers should use ~400X more cells than gRNAs in a screen, to ensure that each gRNA has a good chance to contribute to the screen. In the end, this results in an experiment that could need more than 8x107 successfully targeted cells. Depending on the cells used and the readout, this could be a major limitation.
An alternative to the full genome CRISPR screen approach is to use smaller libraries targeting subsets of genes. With such an approach, you lose the unbiased potential to find things you are not looking for, but there are also benefits gained by lowering the complexity of the screen. For example, the analysis becomes easier, the number of cells needed is lower, and perhaps most important, targeting all genes doesn’t necessarily add value if you are addressing a well-defined, hypothesis driven research question.
Examples of custom CRISPR screens
Differentially expressed genes
As the costs for global gene expression analyses like RNAseq are getting lower and lower, the research community is generating a massive amount of descriptive transcriptomics data. Custom CRISPR screens designed to target differentially expressed genes can be the next stage in making sense of such data. These targeted screens can allow a researcher to test which of the differentially expressed genes found in a gene expression dataset are causal for a phenotype of interest.
Selected pathways
I attended a great talk when I was a PhD student. In particular, one slide of the presentation made a lasting impression on me. The slide showed a complex signaling pathway with many receptors, signaling molecules, transcription factors, and ligands. The presenter then showed which genes they had generated knockout mice for (all of the genes involved as I recall). The lab then used these knockout mice to show exactly which components of the known pathway that were essential for the activity of different ligands. Such knowledge could be very useful for drug discovery, to dissect a complex signaling pathway, and to identify what part of a pathway to inhibit to block the cellular response to a particular ligand (such as when a component of a microbe or a cytokine is added to a cell). With a custom CRISPR screen and the appropriate phenotypic readout, similar mapping could be rapidly performed by targeting all potential components of a signaling pathway. For example, one could study the cellular response to a cytokine known to cause the up-regulation of an important surface protein (e.g. PD-L1 on a cancer cell). In this screen, known components of the cytokine signaling pathway could be targeted. Cells failing to upregulate the protein following stimulation would then be separated by sorting, and subsequently sequenced to identify which signaling molecules were involved in the phenotype.
Surface proteins
My group works on immune cells in different contexts. Most of the immune cells we study are migratory, and their exact localization is important for their function. Their migration is induced by various types of interactions with their environment. These types of interactions to a large extent involve receptors expressed on the cell surface. Thus, a screen can be used to target surface proteins to determine which contact points are involved in a studied cellular behavior. This approach is particularly suitable for in vivo screens, where CRISPR modified cells are transferred into an animal and the behavior of the transferred cells is followed. A benefit of working with surface proteins is also that such proteins are easily studied in living cells using antibodies. Antibodies could further be used to interfere with the identified surface proteins, and such antibodies could thus be interesting drugs.
Known drug targets
Another interesting approach is to design screens against known druggable targets, preferentially those for which validated inhibitors or even approved drugs already exist. If you are lucky and find that a druggable gene has a central role in your system, you could majorly accelerate progression into clinical trials.
Secondary screens
If you have generated a list of candidate genes from a full genome screen, a smaller, secondary, CRISPR screen could be used to validate the list of candidate genes. Green Listed, as of now, contains most of the full genome knockout human and mouse libraries available through Addgene. This means you could perform a screen with one library from Addgene, and then use Green Listed to design a smaller validation screen using selected gRNAs extracted from the same or another library.
These different approaches could also be combined. For example, you could design a screen targeting all differentially expressed genes that are known drug targets, or all differentially expressed genes that code for surface proteins.
One of the first challenges with a custom screen approach is to identify an appropriate list of genes to target. Thus, it is always a good idea to keep a bioinformatician close if you need help putting your list together. Once you have your list of genes, Green Listed can help make the gRNA design process quick and easy.
Green Listed - a CRISPR Screen Tool
Green Listed is a web-based software tool freely accessible though http://greenlisted.cmm.ki.se/. Green Listed can be used to design gRNAs for all the purposes discussed above. Please see videos and text on the website for more detailed descriptions of how to use the software. Here is a brief overview of how the software can be used:
- Design a screen with an easily measureable cellular phenotype (e.g. survival of a cancer cell as a cytotoxic drug is added), and decide which genes you’d like to test for phenotypic effects. Typically the phenotype would be studied in vitro, but there is enormous potential in performing in vivo screens, studying genes affecting cellular behavior in animal model systems.
- Use Green Listed to extract gRNAs that target your list of selected genes. Start by choosing which “Reference Library” you’d like to use in your screen. Currently, eleven published libraries are embedded. These were kindly donated by six different research constellations: Doench/Root (2), Zhang (3), Wu (4), Yusa (5), Wang/Lander/Sabatini (6), and Chari/Mali/Church (7). These reference libraries contain multiple gRNAs targeting all genes in the indicated species and were suggested to be the best gRNAs by the depositing lab. The different labs use different algorithms to calculate which would be the best gRNAs. Interestingly, the libraries do not overlap much, showing that we are far from consensus on optimal gRNA design. With Green Listed you can also easily extract gRNAs for the same gene from several libraries, and identify those overlapping gRNAs that have been “green listed” by several algorithms. Please read the “Detailed Information” text linked to each reference library before using them to understand how they are set up. Using the “User Upload” alternative, you furthermore have the option to upload your own favorite Reference Library, for example related to strategies beyond SpCas9, or species not currently included in Green Listed.
- Provide the list of genes you want to target with your screen in the “Input” box.
- Choose from different “Options”, including adding adapter sequences for cloning purposes.
- Press run and wait for the generation of an output folder containing several files. These include:
Output: A text file containing full information about the suggested gRNAs to use, including all information provided in the original reference library, as well as GC%, reverse compliment sequences, and more.
Output_Compact: A text file that describes which genes were included, and how many gRNAs were identified for each gene. This file can be used to rapidly get an overview of the list of gRNAs generated.
Output_Short: A condensed text file containing a full and a short name for each gRNA, as well as the suggested gRNA sequence including adapters.
Output_UserInputParams: Contains all the information that you have input in the web interface, making it convenient for you to later identify how you were setting up the experiment.
Output_NotFoundList: A text file that appears if genes that you asked for were not found in the reference library. It’s not uncommon that genes have different names, and the software can only extract gRNAs for genes using the name that is used by the selected reference library. If this happens to you, one solution is to identify all the alternative names for your gene of interest (that were not found by Green Listed), and test if any of the alternative names works better. To achieve this you could e.g. copy the list of “Aliases” for your gene from the Wikipedia page of the gene, and paste it into Green Listed.
Note that the output text files can be hard to read. It´s suggested that you open the above text files, copy the content of the file, and paste it into excel. The information then lines up in rows and columns, which are much easier to read.
- Order the suggested gRNA oligos, preferably as an oligo pool. Some companies that synthesize oligos would even accept the Output_Short file directly. We have used CustomArray, Inc for this purpose.
- The gRNA oligos are subsequently cloned into your vector of choice using the adapter sequences that you have specified.
- Run your screen!
Final note
I am very grateful to the researchers letting us use their reference libraries thereby making it possible to put together this software. To me this is a great example of the fantastic openness and generosity of the CRISPR community. It shows how science can be accelerated immensely when we share knowledge and reagents. I have not intentionally excluded any libraries from the software, and my hope is to continue including other libraries. As of now I have focused on human and mouse SpCas9 knockout libraries, but there are of course great libraries targeting other species, and libraries using strategies related to other nucleases as well as inhibitory and activating approaches.
Many thanks to our guest blogger Fredrik Wermeling!
Fredrik Wermeling has a research group at the Centrum for Molecular Medicine (CMM), Department of Medicine, Solna, Karolinska Institutet, in Sweden. He has a particular interest in using molecular tools to study the immune system and cancer. More information can be found through https://wermelinglab.com/.
References
1. Panda, Sudeepta Kumar, et al. "Green listed—a CRISPR screen tool." Bioinformatics 33.7 (2016): 1099-1100. PubMed PMID: 28414855.
2. Doench, John G., et al. "Optimized sgRNA design to maximize activity and minimize off-target effects of CRISPR-Cas9." Nature biotechnology 34.2 (2016): 184-191. PubMed PMID: 26780180. PubMed Central PMCID: PMC4744125.
3. Sanjana, Neville E., Ophir Shalem, and Feng Zhang. "Improved vectors and genome-wide libraries for CRISPR screening." Nature methods 11.8 (2014): 783-784. PubMed PMID: 25075903. PubMed Central PMCID: PMC4486245.
4. Ma, Hongming, et al. "A CRISPR-based screen identifies genes essential for West-Nile-virus-induced cell death." Cell reports 12.4 (2015): 673-683. PubMed PMID: 26190106. PubMed Central PMCID: PMC4559080.
5. Tzelepis, Konstantinos, et al. "A CRISPR dropout screen identifies genetic vulnerabilities and therapeutic targets in acute myeloid leukemia." Cell reports 17.4 (2016): 1193-1205. PubMed PMID: 27760321. PubMed Central PMCID: PMC5081405.
6. Wang, Tim, et al. "Gene essentiality profiling reveals gene networks and synthetic lethal interactions with oncogenic Ras." Cell 168.5 (2017): 890-903. PubMed PMID: 28162770. PubMed Central PMCID: PMC5445660.
7. Chari, Raj, et al. "Unraveling CRISPR-Cas9 genome engineering parameters via a library-on-library approach." Nature methods 12.9 (2015): 823-826. PubMed PMID: 26167643. PubMed Central PMCID: PMC5292764.
Additional Resources on the Addgene Blog
Resources on Addgene.org
- Learn More About CRISPR
- Find CRISPR Screening Libraries
- Browse All CRISPR Plasmids
Topics: CRISPR, CRISPR gRNAs
Leave a Comment